Unleashing the Power of AI Automation
Machine learning (ML) and artificial intelligence (AI) have become transformative technologies that are revolutionizing various industries. ML, a subset of AI, enables systems to automatically learn and improve from experience without being explicitly programmed. Meanwhile, AI encompasses the broader concept of developing machines or systems that can exhibit human-like intelligence and perform tasks that typically require human intelligence. This article explores the intersection of ML and AI, highlighting their key principles, applications, and the potential impact on society.
Understanding Machine Learning
Machine learning is centered around the idea of using algorithms to analyze and interpret large volumes of data, with the goal of enabling machines to make accurate predictions or take informed actions. It relies on three fundamental types of learning: supervised learning, unsupervised learning, and reinforcement learning.
Supervised learning involves training a model with labeled data, where the desired output is known. The model learns to map input features to the correct output by identifying patterns and relationships in the data. This type of learning is commonly used in applications like image recognition, natural language processing, and recommendation systems.
Unsupervised learning, on the other hand, deals with unlabeled data, where the model seeks to discover hidden patterns or structures without any predefined output labels. Clustering, dimensionality reduction, and anomaly detection are common tasks within unsupervised learning, with applications in customer segmentation, fraud detection, and data visualization.
Reinforcement learning involves training an agent to interact with an environment, learning through trial and error to maximize a reward signal. The agent explores the environment, takes actions, and receives feedback in the form of rewards or penalties. This approach has shown remarkable success in tasks such as game playing, robotics, and autonomous systems.
Artificial Intelligence: The Broader Landscape
Artificial intelligence extends beyond machine learning and encompasses a wider spectrum of technologies and concepts. AI aims to create intelligent systems that can perceive, reason, learn, and interact with humans in natural ways. While ML plays a significant role in AI, it is complemented by other fields such as natural language processing, computer vision, expert systems, and knowledge representation.
Natural language processing (NLP) enables machines to understand, interpret, and generate human language. NLP techniques power virtual assistants, chatbots, language translation, sentiment analysis, and text summarization, making human-machine communication more seamless and efficient.
Computer vision focuses on enabling machines to interpret and understand visual information from images or videos. Applications range from object detection and recognition to facial recognition, autonomous vehicles, medical imaging, and quality control in manufacturing.
Expert systems leverage knowledge representation and inference mechanisms to solve complex problems by emulating human expertise. These systems are widely used in domains like healthcare, finance, and cybersecurity, where accurate decision-making based on rules and logic is crucial.
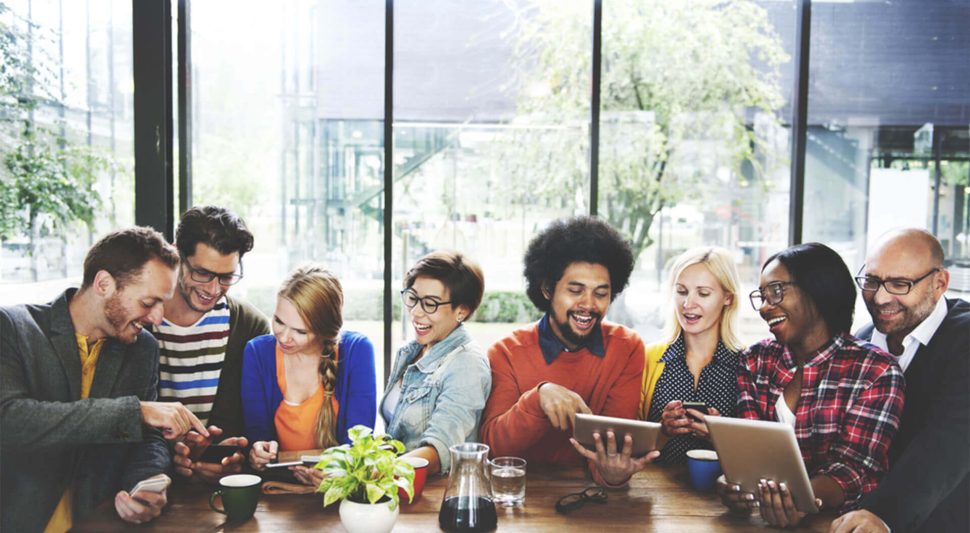
Impacts on Society and Industry
The convergence of ML and AI has immense implications across various sectors. In healthcare, AI-powered systems can assist in disease diagnosis, drug discovery, and personalized treatment plans. AI-driven automation can streamline manufacturing processes, optimize supply chains, and enhance quality control. In finance, ML algorithms can detect fraud, predict market trends, and facilitate risk management. Moreover, AI has the potential to revolutionize transportation, agriculture, education, and many other fields.
However, the rapid advancement of ML and AI also raises ethical concerns. Privacy, bias, accountability, and the potential for job displacement are some of the challenges that need to be addressed. Regulations and responsible AI frameworks are necessary to ensure the fair and ethical deployment of these technologies.
In Conclusion, Machine learning and artificial intelligence are driving significant advancements in technology, enabling machines to perform tasks that were once solely within the realm of human intelligence. ML, with its various learning paradigms, empowers machines to analyze data and make accurate predictions, while AI encompasses a broader set of intelligent systems that interact with humans. As these technologies continue to evolve, their impact on society and industry will only become more pronounced, making it crucial to navigate their development responsibly and ethically.